Interested in learning more about Customer Lifecycle Scoring from Correlated? Sign up here to watch a live demo.
Getting account scoring right can significantly improve the efficiency of your business by allocating resources towards the best accounts. But what is it, why do you need it, and why is it so hard to build lead scoring models the right way? In the following blog post, we’ll dive into these topics and more, read on!
What is account scoring and why should I care?
Account scoring is essentially the act of collecting data about a company and their behavior to assign a predictive score that measures the propensity that company has to spend money on the product.
Account scoring is literally everywhere. In B2B SaaS, account scoring is most noticeably used in the marketing realm, where marketers score inbound website traffic to identify quality leads that could convert to customers. These qualified accounts are passed on to sales as they are predicted to have a higher propensity to convert.
Account scoring is everywhere because it’s a powerful concept with clear, positive business impact. By leveraging the data you’re collecting about potential customers, you can identify, prioritize, and target which customers to go after, resulting in a much more efficient go-to-market engine. Rather than wasting time on prospects who simply aren’t ready to buy, you can focus your efforts on prospects more likely to convert. Less wasted time = less wasted money = lower acquisition cost = better revenue multiples.
Accounts can and should be found across the entire customer lifecycle, not just at the top of the funnel
With the advent of SaaS, software is now hosted in the cloud, enabling companies to collect more first-party data to measure intent. This data can be used in conjunction with traditional third-party data to further improve account scores.
Even more importantly, SaaS products involve annual subscriptions that can be expanded or lost through churned customers. Whether it’s prior to purchasing or after a purchase, every single user interaction can have an impact on your bottom line. The concept of account scoring can and should be applied throughout the customer lifecycle to optimize go-to-market not just at the top-of-funnel with MQLs, but throughout the funnel as well.
Laying the foundation for scoring your customer base could not be more important!
Account scoring vs. lead scoring
Isn't account scoring the same as lead scoring? Yes and no. Traditionally in B2B SaaS, you would score inbound leads based on criteria related to an individual's actions on the website, how they answered form fill questions and whether they worked for a company in your ICP.
This approach is still used today and it's commonly referred to as marketing qualified leads (MQLs). This is an effective approach for qualifying hand raisers and other individuals that show some signs of engagement with your website content.
The problem with MQLs and traditional lead scoring is that in B2B, you often sell to companies not individuals. Whether or not a person signs up for a whitepaper or starts using your free product are signals that should be incorporated into a score that relates to the company they work for. What you really want to know as a salesperson is the answer to "what accounts should I be targeting right now." That's where account scoring comes in.
The two most common approaches to account scoring
Hopefully we convinced you that account scoring is a powerful and useful tool to superpower your go-to-market. But how do you get started? Let’s go through the two most common approaches SaaS businesses take when it comes to account scoring.
Approach 1: Rule-based / weighted scoring
Perhaps the most common approach when it comes to account scoring is rule-based or weighted scoring. This approach involves assigning weights or points to certain actions or traits in order to qualify an account or lead. An important thing to recognize about rule-based scoring is that it isn’t really predictive, rather it seeks to identify customers who best fit the profile of customers you expect to convert.
A simple example might be something like the following:
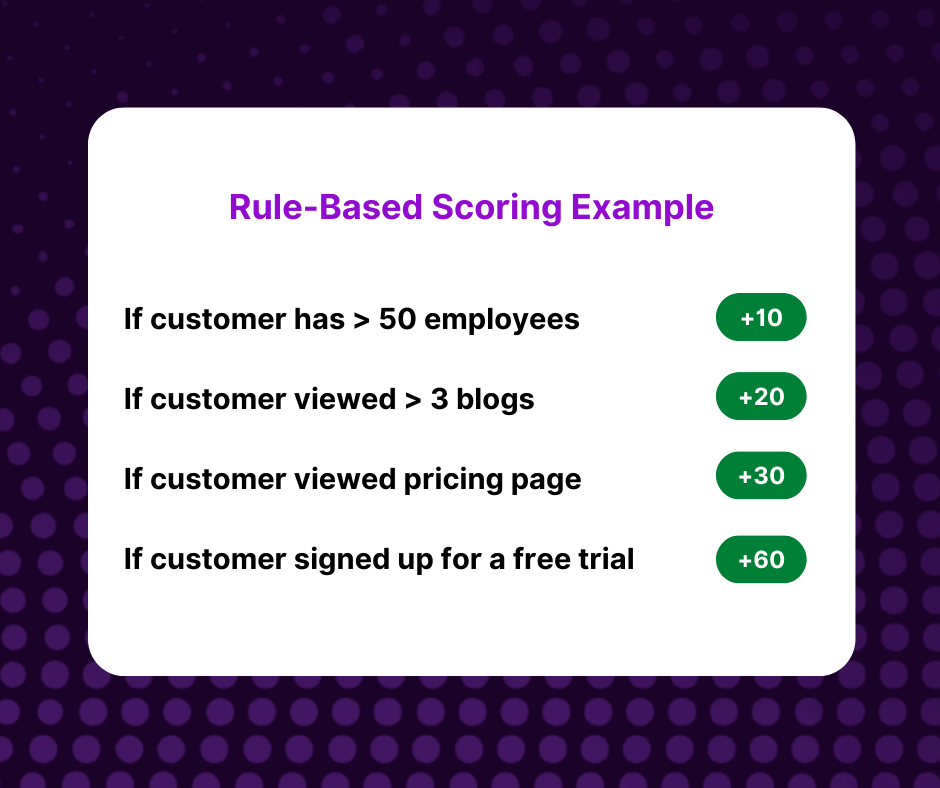
Customers with a score greater than 50 are considered qualified.
Hubspot and Marketo provide solutions that enable this approach to score marketing qualified leads. They don't really offer account-based solutions unless you want to pay extra in the case of Marketo.
Approach 2: Propensity models using machine learning
A more advanced approach is to leverage machine learning to predict a user or account’s propensity to buy. This is typically done by data science teams and can take months to implement for the first time. Subsequent adjustments to the scoring model can similarly take months.
Despite the high investment required, many companies are building propensity models simply because understanding which customers to talk to when they are ready to convert is mission critical.
There are several different machine learning models that can be used to predict propensity to buy. You can consider using binary prediction models like logistic regression or boosted trees, or leverage clustering models to identify look-a-alike audiences.
A more trivial, but no less effective method to score accounts is to leverage your data science team to identify shared traits across converted users and compare those with shared traits across non-converted users. You can then use these insights to build your own rule-based or weighted scoring model.
Which approach is better?
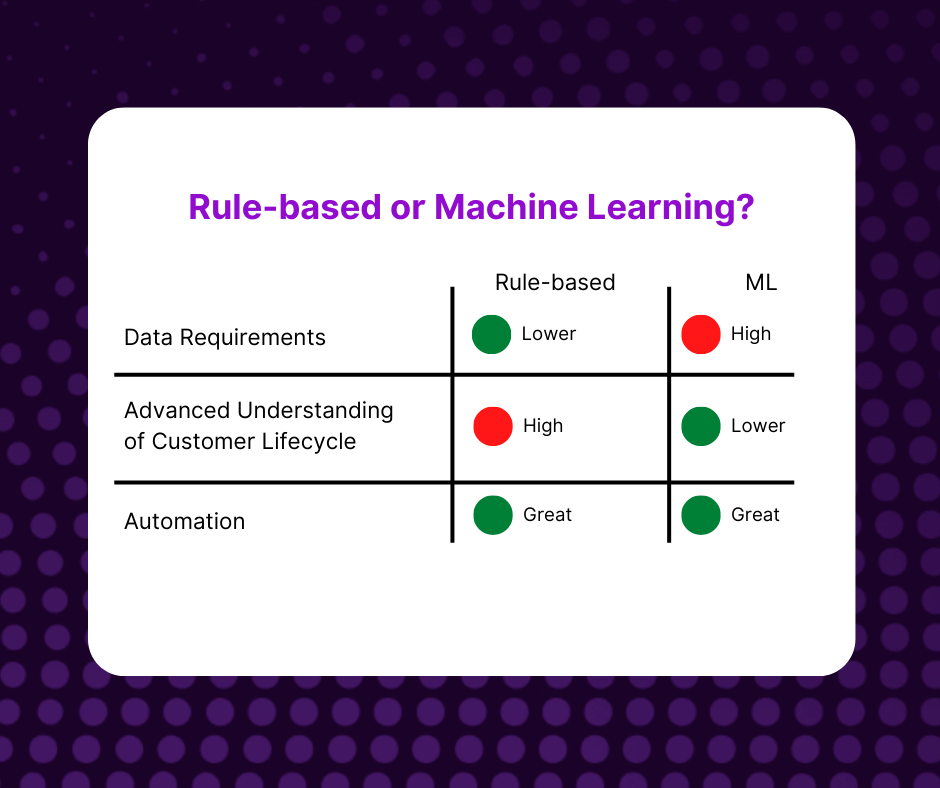
When you’re thinking about which approach to implement, it’s important to consider several criteria to choose the approach that fits your company’s unique needs.
Do you have enough data to leverage machine learning effectively?
Machine learning is heavily influenced by the data you use to train your models. You must have a decent amount of data, as well as enough positive examples of conversions, to output a model that performs well. If you have less than 100 positive data points, it’s likely that rule-based scoring will work better for you to start.
Do you have enough understanding of what goes into your score to assign appropriate rules and weights?
One of the biggest weaknesses of rule-based / weighted scoring is that it relies on what you know about your customer base. First, you need to believe that you have a good understanding of what drives conversion. Second, you have to understand how to weigh those factors appropriately. At the end of the day, we’ve noticed that many companies end up with a pretty arbitrary scoring mechanism. The benefit of machine learning is that you allow the probabilistic model to choose the right weights for the data points you have. Although you may not have as much manual control, the insights on which you are basing that control might be skewed anyway. Further, machine learning can help you identify trends you didn’t know about, thus expanding the insights you leverage to build out your own score.
Who is using the score and what context do they need to use it?
Are you expecting to fully automate outreach (e.g. sending drip campaigns to qualified leads), or are you expecting a human to reach out (e.g. assigning sales reps to the best leads)? If you’re looking to fully automate outreach, a machine learning generated score will be a good start when it comes to prioritize who receives an email. However, if you’re sending leads to a sales rep for personalized outreach, the sales rep will need more context in order to act on the best leads.
That’s when explainability becomes important. With rule-based and weighted scoring, because you’re defining the criteria manually yourself, you’ll be able to pass along those data points when you pass along the score. On the flip side, machine learning models do provide some insight into how scores are weighted, but ultimately, the exact driving factors behind a specific score are more fuzzy. At Correlated, we surface driving factors behind scores, but it’s important to note that machine learning models are probabilistic and that many factors can drive a score beyond the highest weighted factors.
Ultimately, we believe that a combination of both is the best path forward in account scoring. First, you use machine learning scoring to cast a wide net across all your customers to identify leads. Second, rather than using rule-based or weighted scores based on arbitrary rules and weights, you can define specific “signals” based on your own domain knowledge to further capture additional leads. For example, you can create a conversion score to identify the leads most likely to convert, sending them through a drip campaign. You can then set up custom signals to identify which users tried 3 of the 5 paid features, and pass those along to a sales rep.
By mixing and matching both approaches, you can get the best of both worlds: capturing the accounts you know are good based on your domain knowledge, and the accounts you didn’t know were good using machine learning. Correlated supports both these approaches!
Account scoring powered by machine learning is hard to get right
It’s important to understand how important data quality is when it comes to leveraging machine learning for propensity scoring. Although you can throw anything at a model, anything can also come out. That’s why it’s important to consider what data you’re including in the model. Let’s go through some common data quality issues that can skew your model results.
Having too many unique values for a given feature
The next time you’re chatting with your data science team, ask them about the cardinality of your features. This basically means the total number of unique values a given feature has. For example, let’s say you have the job title for every single user. But, instead of using a picklist, you had everyone type in their job title. Now that column has every permutation under the sun of “software engineer” as a job title.
In this case, you have a lot of unique values for job title. Basically, you have a high cardinality problem! The problem with machine learning models is that they don’t know the meaning behind job titles, so they will interpret “Software Engineer” as different from “Software Engineer I”. This can cause problems with certain machine learning models and impact the results.
Including too much missing data
Here’s another way to describe this to your data science team if you want to speak their language: sparse data. Sparse data basically means that you are giving the machine learning model data that is missing a lot of fields. This can cause problems where accounts or users who have values for fields are given a better score, despite the field itself not having a positive impact on the results (or vice versa).
Helping the machine learning model cheat
Finally, you want to make sure that you’re not giving your machine learning model data that helps it cheat. This typically means that you’re giving it something that is too correlated to the goal, resulting in predictions that aren’t really leveraging leading indicators.
Here’s a common example: let’s say that you’re trying to find users who convert, and you’ve defined that as having a non-null value for their Plan Type. However, you also gave the machine learning model ARR as a data point to use. Well obviously, if ARR is greater than 0, the user converted. That’s helping the machine learning model cheat! You’ll end up with a highly accurate machine learning model that performs well on training data, but fails in practice.
How do you know if account scoring is working?
Well of course, the obvious answer is to measure conversions. Two measurements that are useful are:
- The percentage of qualified accounts who converted.
- The percentage of all conversions that were marked as qualified.
But perhaps a more important measure of success is qualitative, not quantitative. One of the biggest challenges that companies encounter when putting in place an account scoring strategy is operationalizing that strategy. They’ll come up with a lead score that sales reps don’t understand, or that GTM teams don’t trust enough to use in practice.
That’s why it’s important to not only measure the data, but also to get qualitative feedback from downstream teams on how they are receiving and utilizing the scores.
If you found this article interesting and are curious about expanding your scoring use cases beyond MQLs, we invite you to check out Correlated. Correlated offers a Customer Lifecycle Scoring platform that allows you to create custom machine learning models to score both accounts and individual users. We cover the entire lifecycle, from onboarding to conversion to expansion to churn, allowing you to make your entire GTM process more efficient by laying on AI.
Next Steps with Correlated
We offer a free trial for 30 days. Get started today!